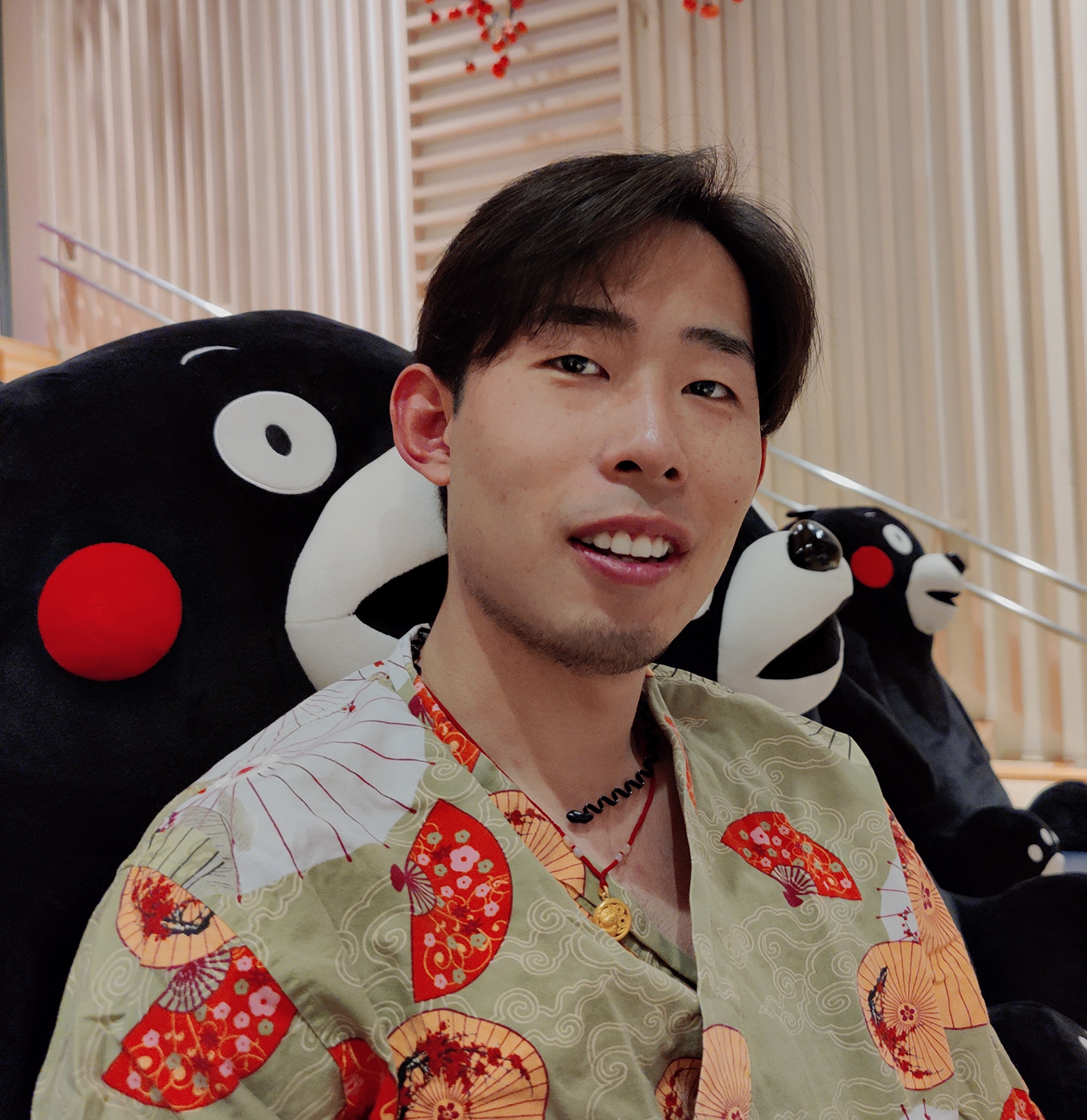
Ph.D. Candidate at Fudan University
About Me
I am a Ph.D. candidate in the Collaborative Information and Systems Laboratory (CISL) (opens new window) , School of Computer Science at Fudan University, advised by Prof. Ning Gu (opens new window).
My research interests include Human-centered News Recommendation, Computer-supported Collaborative Works (CSCW) & Social Computing, and Human-computer Interaction (HCI). I graduated from Shanghai University of Finance and Economics (SUFE) in 2020 with a B.Eng. in Computer Science.
News
- 📌 [04/2024] We've released the Code Repo (opens new window) of our TCSS paper "Simulating News Recommendation Ecosystems for Insights and Implications" (opens new window)
- 📌 [04/2024] We've released the Code Repo (opens new window) of our TWEB paper "Heterogeneous Graph Neural Network with Personalized and Adaptive Diversity for News Recommendation" (opens new window)
Education
-
Ph.D. Candidate, School of Computer Science, Fudan University. 2020.9 - Present
Advisor: Prof. Ning Gu (opens new window)
-
Teaching Assistant - Social Computing (Undergraduate Course)2024.2 - 2024.7
Advisor: Prof. Tun Lu (opens new window)
-
Teaching Assistant - Social Computing (Graduate Course)2022.2 - 2022.7
Advisor: Prof. Tun Lu (opens new window)
-
Bachelor's Degree (with honor), School of Information Management and Engineering, Shanghai University of Finance and Economics. 2016.9 - 2020.6
GPA:3.8/4.0 | Ranking: 1/67
Research Interests
- Human-centered News Recommendation
- Computer-supported Collaborative Works (CSCW) & Social Computing
- Human-computer Interaction (HCI)
Publications

-
Guangping Zhang, Dongsheng Li, Hansu Gu, Tun Lu, Li Shang, Ning Gu.
Studying the evolution of online news communities is essential for improving the effectiveness of news recommender systems. Traditionally, this has been done through empirical research based on static data analysis. While this approach has yielded valuable insights for optimizing recommender system designs, it is limited by the lack of appropriate datasets and open platforms for controlled social experiments. This gap in the existing literature hinders a comprehensive understanding of the impact of recommender systems on the evolutionary process and its underlying mechanisms. As a result, suboptimal system designs may be developed that could negatively affect long-term utilities. In this work, we propose SimuLine, a simulation platform to dissect the evolution of news recommendation ecosystems and present a detailed analysis of the evolutionary process and underlying mechanisms. SimuLine first constructs a latent space well reflecting the human behaviors and then simulates the news recommendation ecosystem via agent-based modeling. Based on extensive simulation experiments and the comprehensive analysis framework consisting of quantitative metrics, visualization, and textual explanations, we analyze the characteristics of each evolutionary phase from the perspective of life-cycle theory and propose a relationship graph illustrating the key factors and affecting mechanisms. Furthermore, we explore the impacts of recommender system designing strategies, including the utilization of cold-start news, breaking news, and promotion, on the evolutionary process, which sheds new light on the design of recommender systems.

-
Guangping Zhang, Dongsheng Li, Hansu Gu, Tun Lu, Ning Gu.
The emergence of online media has facilitated the dissemination of news, but has also introduced the problem of information overload. To address this issue, providing users with accurate and diverse news recommendations has become increasingly important. News possesses rich and heterogeneous content, and the factors that attract users to news reading are varied. Consequently, accurate news recommendation requires modeling of both the heterogeneous content of news and the heterogeneous user-news relationships. Furthermore, users' news consumption is highly dynamic, which is reflected in the differences in topic concentration among different users and in the real-time changes in user interests. To this end, we propose a Heterogeneous Graph Neural Network with Personalized and Adaptive Diversity for News Recommendation (DivHGNN). DivHGNN first represents the heterogeneous content of news and the heterogeneous user-news relationships as an attributed heterogeneous graph. Then, through a heterogeneous node content adapter, it models the heterogeneous node attributes into aligned and fused node representations. With the proposed attributed heterogeneous graph neural network, DivHGNN integrates the heterogeneous relationships to enhance node representation for accurate news recommendations. We also discuss relation pruning, model deployment, and cold-start issues to further improve model efficiency. In terms of diversity, DivHGNN simultaneously models the variance of nodes through variational representation learning for providing personalized diversity. Additionally, a time-continuous exponentially decaying distribution cache is proposed to model the temporal dynamics of user real-time interests for providing adaptive diversity. Extensive experiments on real-world news datasets demonstrate the effectiveness of the proposed method.
Awards & Honors
- 2020 The 1st Place in the ChineseCSCW 2020 Big Data Challenge (opens new window)
- 2020 Outstanding Graduate of Shanghai
- 2018 Chinese National Scholarship